Refine or Rebuild: When Your ML Algorithms are Under Disruption
Disruptions at the global scale seem to be a norm today. We are seeing everything from the pandemic, leading to supply chain restructuring, to war in Ukraine, leading to energy and food crises, interest rate hikes, and financial instability. These global disruptions are forcing many to reconsider how they use complex algorithms and models for forecasting a variety of tasks – from sales to budgets to when certain items come back in stock. These are unprecedented times, so it’s no surprise that historical data is no longer a strong predictor of the future, although they are still useful.
Many have debated whether it’s best to tune and adjust existing models trained with historical data, or completely rebuild with a better understanding of today’s “new normal.” The answer will depend on how adaptive your models are and how fast they can learn from the recent data. Before making potentially business-altering decisions that could impact the customer experience, leaders need to take a step back and make sure they understand a common misconception: the difference between machine learning (ML) models and true AI models.
Understanding the Difference Between Static Machine Learning and True AI
It’s natural to want to draw causal conclusions from correlated events. Unsurprisingly, many believe “their forecasting models have stopped working” is due to the unforeseen circumstances from COVID-19. In reality, the more common cause of this has to do with the type of model used. More often than not, teams use ML models that do not learn constantly, as opposed to a true AI model, which can learn and refine its model continuously.
Making the distinction between ML and AI is key here: machine learning is only a part of AI, just as an engine is only a part of a car. ML is not a kind of AI just as an engine is not a kind of car. A true AI model is capable of incorporating feedback data through the learning loops to update the model every time it’s used. Although ML models can be updated, this doesn’t happen automatically. Data scientists are required to collect more data to re-train and refine the model. Using true AI should give you a much better chance of adapting to current situations (along with some manual tuning).
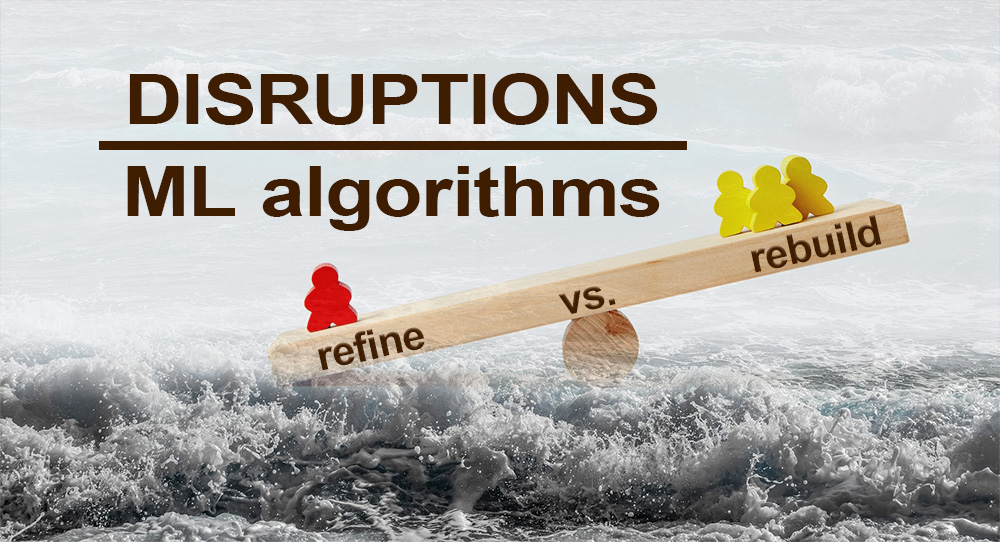
Making the Transition from Machine Learning to AI
Now you may be thinking: I’m using a ML model. How can I turn this into a true AI model? There are 3 steps business leaders need to consider as they make the leap from static ML models to true AI.
Step 1: Collect feedback and outcome data to complete the learning loops. For example, historical weather data can be used to predict the current weather, but without feedback data on how good its prediction is, it will be challenging for the model to course correct and refine itself to improve its performance automatically. Feedback data is crucial for the model to learn and adapt.
Step 2: Leverage feedback data to refine the model. If final outcome data isn’t collected in the first place, it’s unlikely that your current model is equipped to leverage this data as input. Collecting the feedback is one thing. Now, your data scientists will have to modify the model to take advantage of this feedback data to improve the model. It makes sense intuitively, but there are lots of technical work here for your data science and data engineering teams.
Step 3: Make the ML process more dynamic and automatic. The traditional ML process in an enterprise relies on data scientists to collect a new batch of data over a period of weeks or months, and then use it to retrain the model. By automating the model update process, the operating model can be updated more frequently, learn from new data, and adapt to new market conditions much faster.
Common Challenges During the Transition
Each step in the transition to true AI poses challenges in different parts of the business.
Step 1: Overcoming business limitations is one of the biggest challenges to collecting feedback and outcome data. Companies often don’t collect larger amounts of data because it’s either expensive, or they don’t know how it can be used. Outcome and feedback data is crucial for AI, but are not often seen as mission-critical for business. While this data is useful to refine the model, it’s not needed for the model to operate under stable business conditions. Convincing business leaders to invest in instrumenting a new feedback collection mechanism and all the data science and engineering required for the proper usage of this data is usually a huge uphill battle.
Step 2: Most data science challenges occur when teams try to leverage feedback data to refine models as part of the transition to true AI. Data scientists have to extract the information from the feedback data and use it to refine and update the model. The model then needs to be revised to take feedback data as a standard input to the model. Although this sounds difficult, there are standard procedures and best practices in statistics and ML to accomplish this, as long as you have good data scientists on board.
Step 3: The final step, making the ML process more automatic, has its own challenges, though these are mostly engineering and operational challenges. These include engineering the feedback data pipeline to handle the speed and scale at which feedback data is generated, and making the ML engine more dynamic by giving it sufficient computing resources to retrain, update and redeploy the model in a timely fashion. When either part becomes a bottleneck, our model won’t be updated as frequently as we want, and therefore the model will not be able to adapt fast enough to the dynamic market condition.
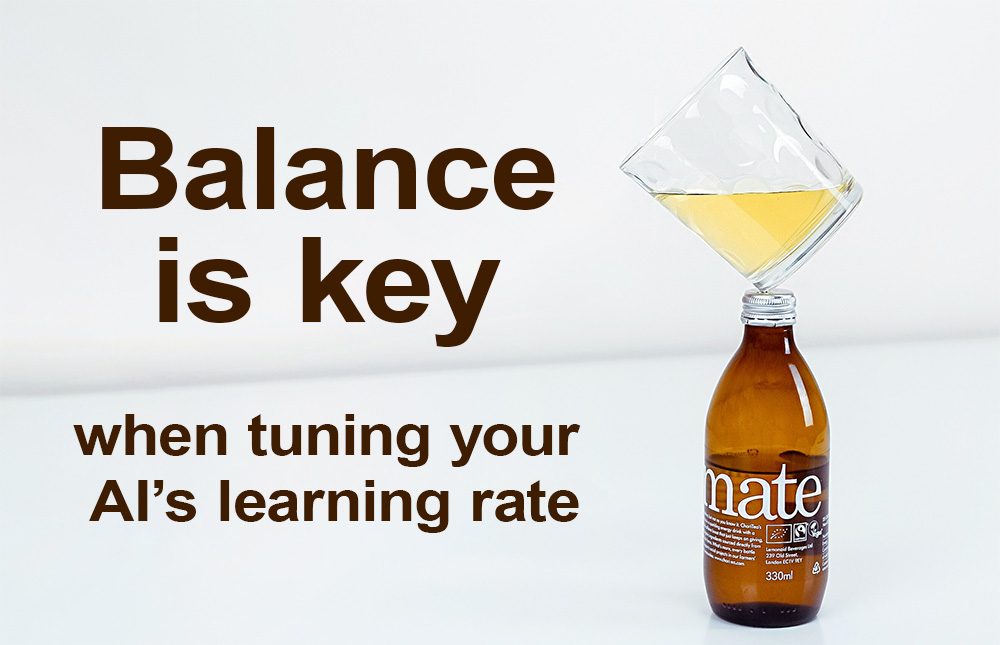
The Balancing Act of Tuning the Learning Rate
Once the transition to true AI has been made successfully, your model should be able to learn from new data and adapt. However, this doesn’t mean your AI will automatically adapt to current market conditions well. Learning takes time, and an AI’s learning rate will determine how fast it adapts. The AI wouldn’t be able to adapt fast enough if its learning rate is too slow, but we can’t just arbitrarily increase the AI’s learning rate either. If the learning rate is too fast, our AI’s output will become noisy and unstable, and therefore suboptimal. Setting the proper learning rate for our AI is a balancing act. That’s why a bit of manual tuning is still required when the AI is in shock due to severe market disruptions.
Conclusion
The final question remains, “Since historical data is no longer representative of the current situation, should we rebuild our model?” Even with the proper learning rate for our AI and its ability to learn from recent data, why keep the history if it’s not helping? My response to this would be “don’t be so short-sighted.” Just because the history is not useful now, doesn’t mean it won’t be useful in the future. If you get rid of it now, there is no turning back even when you do find a use for it in the future.
Refine the model instead of rebuilding it from scratch. One of the biggest lessons learned from the pandemic is that businesses need to be agile to weather unforeseen crises. Companies need to arm themselves with the digital tools and processes that can make agility happen – and transitioning from static ML to true AI models will help. If history is indeed completely useless, our feedback data will tell us that through the learning loop and make our model ignore it. So, it never hurts to keep the historical data.
At last, turbulence will calm, and we will reach a new normal where the relevance and usefulness of our history could increase. Take these steps now to transition to a true AI model and future-proof your business for the next unexpected turn.
DISCLAIMER: The content of this article was first published by CMS Wire. Here is the latest revision and a current rendition of the raw manuscript submitted to the publisher. It differs slightly from the originally published version.